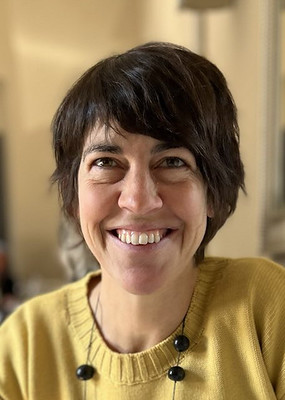
“Turbulent predictions and navigation with odor time traces”
In this talk I will discuss computational models of olfactory predictions and navigation using turbulent odor cues. I will ask whether effective strategies can be learned as a response to short excerpts of odor time traces, with no prior information on the odor cues nor spatial perception. First, I will use kernel methods to show that sensing for as little as a few seconds is sufficient to predict robustly where an olfactory target is. The longer the memory the better the predictions. Robust predictions are achieved by combining the complementary information from intensity and sparsity of the odor. Second, I will use these features to construct few discrete olfactory states. I will show a model-free reinforcement learning algorithm that learns navigation as a response to these olfactory states, with no prior information on the odor nor spatial maps. An optimal memory for navigation emerges due to the need to negotiate between following the cues and recovering the plume when no odor is detected. I will discuss the connection of memory with the physics of turbulent odor cues.
Hosts: Thierry Emonet and Michael Abbott