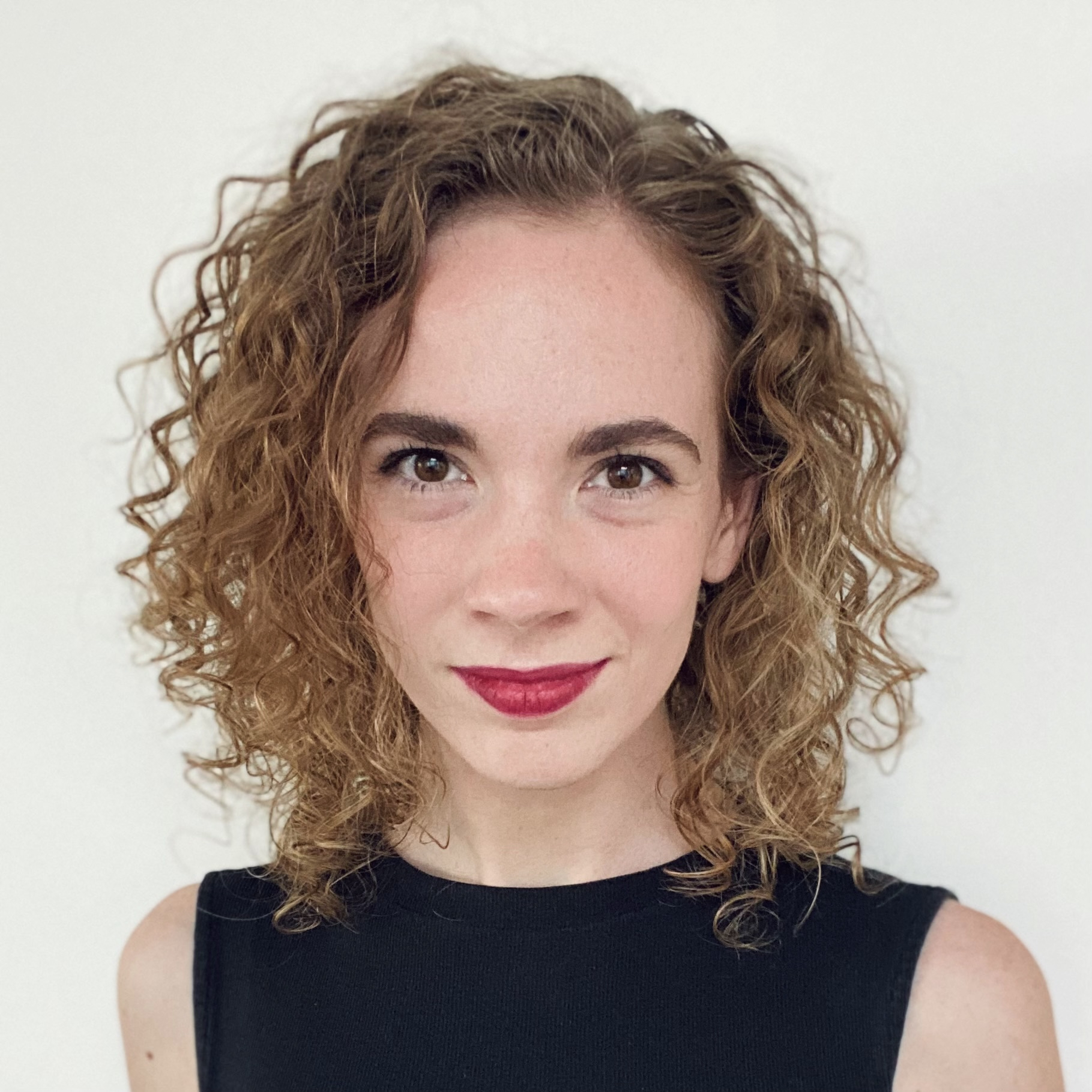
On Multimodal Embeddings for Scientific Data
Scientific datasets are remarkably diverse – for instance, particle collision events at the LHC, weather forecasts, and protein folding simulations are all fundamentally quite different in both information content as well as structure. Unlike most industry applications, in which multimodal models typically handle only a handful of modalities at a time, scientific applications can easily comprise dozens of modalities. This diversity poses an interesting challenge for ML applications: how can we build joint embedding spaces that meaningfully represent such heterogeneous data? When is this beneficial for science, and when might it hinder performance? In this talk, I’ll discuss some emerging strategies for embedding diverse scientific data types into unified spaces. I’ll discuss the potential benefits of these shared embeddings for scientific analyses as well as some of their practical and theoretical drawbacks.
Host: Nathan Suri & Naomi Gluck